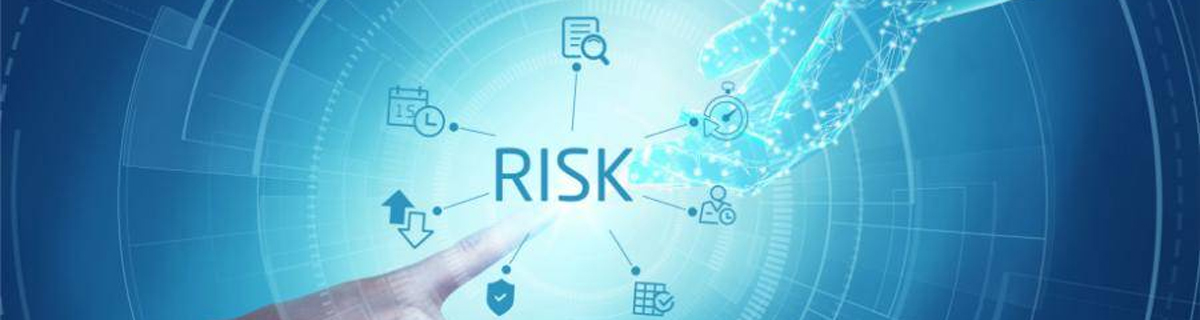
The model is based on the large-scale sampling data of the target group, dig out the phenomenon nature and operation law of a practical problem or objective thing, use abstract concepts to analyze the existing problems or risks, calculate and deduce the countermeasure process of reducing and preventing problems or risks, and form a set of systematic strategies or rule set. The risk control model refers to a model that evaluates and controls risks by using data analysis and algorithm technology in finance, insurance, e-commerce and other fields. It is a mathematical model built based on a large number of historical data and statistical methods to help institutions or enterprises to effectively identify, quantify and manage the risks associated with their business, so as to reduce economic losses and improve operational efficiency.
Risk identification: The risk control model can identify potential risk factors and abnormal patterns through statistical analysis and data mining methods, so as to help institutions to find and warn risks in time.
Quantified risk: The risk control model can quantify the risk, and calculate the probability and probability of the risk, influence degree and loss estimation, so as to provide scientific basis for decision-making.
Control risk: Risk control model can establish the risk assessment model and decision model, and formulate the corresponding risk management strategies and control measures to help institutions reduce the possibility of risk occurrence and the degree of loss.
Improve efficiency: The risk control model can automatically and process a large number of complex risk data and decision-making process in a standardized manner, improve the efficiency and accuracy of risk management, and reduce labor costs and error rate.
Risk: What are the main risk models?
According to different application scenarios and methods, the risk control model can be mainly divided into anti-fraud model, risk prediction model, score card model, credit model and arbitrage risk model.
Anti-fraud model: Fraud detection model analyzes user behavior patterns and abnormal behavior characteristics, and uses machine learning and data mining algorithms to identify and predict potential fraud.
Risk prediction model: Market risk model models and analyzes market price fluctuation, systemic risk and return on investment by modeling and analyzing financial market data.
Score card model: The scoring card model is based on statistical methods and machine learning algorithms. By analyzing a large amount of historical data, the customer credit score card is built to predict the default probability and credit rating of customers.
Credit model: Credit risk model is used to evaluate and manage the credit risk of borrowers. It predicts the possibility of default and loss probability by analyzing the financial status, historical credit records and other data of individuals or organizations.
Arbitrage risk model: The arbitrage risk model identifies arbitrage opportunities and risks by analyzing the price differences and trading rules of different markets or products, and provides a basis for investment decisions.
Different enterprises have different business scenarios, different process standards and demand objectives, and the direct use of the general standard model is often not satisfactory. Therefore, the types of risk control models may vary in different industries and scenarios, and some models may also be constructed and applied in combination with various methods and technologies.
Dinsight: Step-by-Step Model Management for Decisioning Engine
decisioning engine is the core system for managing and executing risk control models and is responsible for integrating, deploying and monitoring the operation of various risk control models. Based on actual business requirements, performance and stability requirements must be considered to achieve efficient and reliable risk management.
How does the risk control engine manage the model? Dinsight provides a visual interface that lets business people get started quickly, easily query, import models, and observe model status in real time.
Log in to Dinsight background, go to "Variable Management - Model Management" and click on "Model Warehouse". In the current page, enter the model name, model ID, model state, etc., you can filter the query according to the type of model.
Under this page, click the "Model Import" button in the upper right corner, and the system popup box to select the model file to be uploaded. The top image Dinsight real-time risk control engine supports PMML model, POython model and platform standard model.
After importing the model file, you can view the input and output parameters in the model in time, and the output parameters can be used in the policy. Click OK to complete the import operation and view the new model in the Model Warehouse.
Statistics on model service monitoring, stability monitoring, and performance monitoring can be viewed under the Model Dashboard. Select any model, and the system will show the relevant data indicators of the model.
When the model is used by the strategy, you can see today's call amount and time consumption to be used in this module. Detailed indicators such as historical call amount trend statistics, historical performance trend statistics, model stability and score distribution can be viewed according to different time dimensions.
Dinsight can perform risk judgment on the requests sent by the business front end in marketing activities, payment orders, credit applications and other scenarios, and return the decision result within milliseconds to improve the risk prevention and control ability of the business system. The average processing speed of daily risk control strategies is less than 100 milliseconds, the aggregate data engine, the integration of expert strategies, the parallel monitoring, replacement and upgrading of existing risk control processes, and the construction of a dedicated risk control platform for new businesses; Aggregate anti-fraud and risk control data, support multi-party data configuration access and precipitation, can be configured graphically, and quickly applied to complex strategies and models; Based on the experience reserve of mature indicators, strategies and models, as well as deep learning technology, self-performance monitoring and self-iteration mechanism of risk control can be realized; Integrated expert strategy, based on system + data access + index library + strategy system + expert implementation of actual combat; It supports parallel monitoring, replacement and upgrading of existing risk control processes, and can also build a dedicated risk control platform for new businesses.