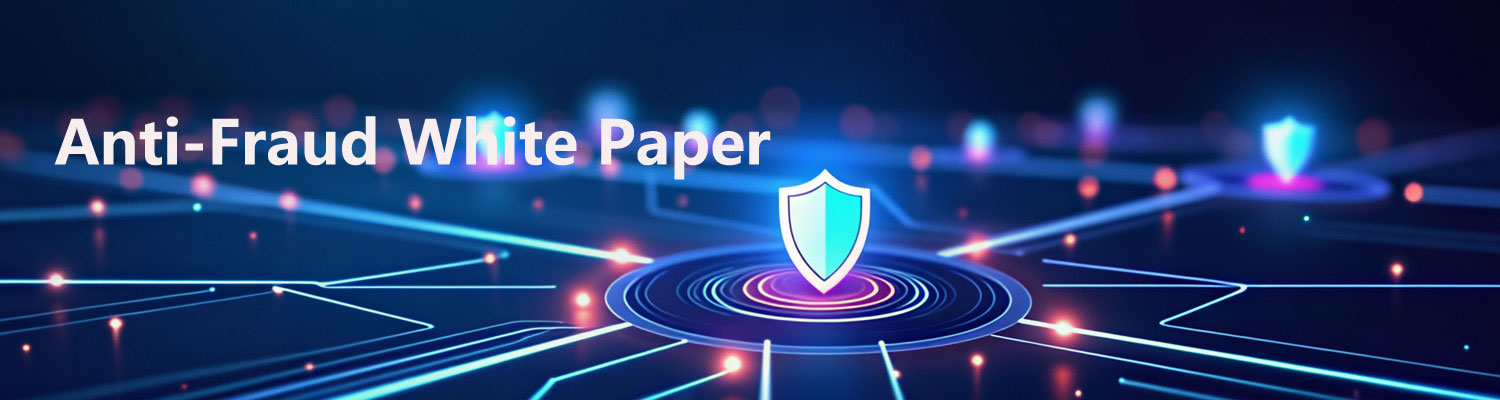
Recently, at the "Digital Intelligence Empowerment: Financial Technology Driving High-Quality Development in Finance" forum hosted by Bank of Communications, the Financial AIGC Audio-Video Anti-Fraud White Paper(Click here to download) was released. Jointly authored by Bank of Communications, Dingxiang Technology, and RealAI, this white paper systematically explores the risks and challenges brought by AIGC (AI-generated content), focusing on the audio-video fraud challenges faced by the financial industry. It serves as a reference for financial institutions to enhance their capabilities in identifying and preventing AIGC-related fraud. The white paper provides detailed insights into professional AIGC audio-video detection technologies and products designed to combat AIGC audio-video fraud.
AIGC Audio Forgery Detection Technology
The core objective of AIGC audio detection technology is to improve accuracy and reliability in the face of continuously advancing audio forgery techniques, focusing on audio quality, voiceprint features, and spectrum analysis.
Audio Quality: Forged audio often exhibits quality anomalies, such as noise, distortion, or other imperfections that affect clarity. Since the process of generating synthetic audio typically introduces these unnatural elements, they serve as important clues for detecting fake audio.
Voiceprint Features: Each person’s unique vocal characteristics, shaped by their physiological traits like vocal cord structure and speaking habits, create distinct voiceprints. AIGC-generated audio often lacks the personalized nuances of human speech, tending to be overly regular and mechanical in aspects like tone and pitch, which forms the basis for voiceprint detection.
Spectrum Analysis: Spectrum analysis converts audio signals from the time domain to the frequency domain to examine frequency components. In audio forgery detection, AIGC-generated audio often exhibits unnatural features in high or low-frequency ranges, such as irregular frequency distribution. These anomalies can be revealed through spectrogram analysis.
AIGC audio detection technology combines multi-level feature fusion, adversarial training, and temporal modeling to maintain high-precision detection performance even in the face of various generation techniques and complex noise interference. Looking ahead, the integration of multimodal information and more advanced deep learning technologies is expected to further improve the detection of forged audio.
AIGC Image Forgery Detection Technology
The primary task of AIGC image forgery detection is to determine whether an image was generated or tampered with by artificial intelligence. Evidence of forgery is typically reflected in visual artifacts, digital signal anomalies, model fingerprints, facial priors, and violations of physical imaging principles.
Visual Artifacts: AIGC image generation may produce unnatural visual effects caused by algorithmic limitations, insufficient training data, or computational constraints. For example, generated images might display unclear or distorted details.
Digital Signal Anomalies: Forged images may show irregularities in frequency, noise, or color statistics. In the frequency domain, AI-generated images may exhibit specific patterns, such as artifacts introduced by upsampling operations. In the noise domain, generated images often have noise patterns distinct from those in real images. These signal-level anomalies can be analyzed to detect forged images.
Model Fingerprints: The process of AI-generated images leaves unique “model fingerprints,” determined by the architecture, training data, and parameter settings of the generative model. Specialized detection models can identify these fingerprints to determine whether an image was generated by a specific model.
Facial Priors: In generated facial images, anomalies such as misaligned eye positions, irregular pupil shapes, or unnatural nose structures may appear. These issues often indicate the image was AI-generated.
Physical Imaging Principles: Real images adhere to physical rules of light propagation, reflection, and refraction. In contrast, AIGC-generated images may exhibit phenomena that violate these rules, such as unnatural shadow directions or incorrect perspective relationships.
Methods for detecting forgery clues in AIGC images can be broadly divided into handcrafted approaches and representation learning. Handcrafted methods design specific features based on an understanding of forgery mechanisms to identify traces of manipulation. Representation learning, on the other hand, leverages deep learning to handle the complex and evolving challenges of image forgery in an automated and efficient manner. Although representation learning offers adaptability and efficiency, handcrafted methods remain irreplaceable in certain scenarios due to their transparency and interpretability.
AIGC Video Forgery Detection Technology
The core objective of AIGC video detection technology is to accurately determine whether video content is AI-generated or tampered with. Compared to image forgery detection, video forgery detection involves not only clues present in static images but also dynamic features such as temporal visual artifacts, audiovisual inconsistencies, and the naturalness of motion trajectories.
Clues to forgery in AIGC videos include static image forgery clues as well as temporal and dynamic variations in the video. Temporal visual artifacts refer to unnatural transitions between consecutive frames in a video, often manifested as frame jumps or discontinuous changes. Audiovisual inconsistencies arise when the visual and auditory information in a video are not perfectly synchronized, particularly in generated videos where sound fails to align with the visuals. The naturalness of motion trajectories is another key clue. In AI-generated videos, object movements may appear rigid, jerky, or physically implausible, such as abrupt speed changes or abnormal acceleration.
Modeling methods for detecting forgery clues in AIGC videos can be categorized into handcrafted approaches and representation learning.
Handcrafted Feature Modeling:
This approach relies on traditional "feature engineering," focusing on video-specific attributes. For example, techniques such as optical flow and trajectory tracking can analyze object motion trajectories within a video to detect unnatural movement or abrupt speed changes. Additionally, analyzing spatiotemporal consistency between video frames, such as the coherence of color, brightness, and texture across consecutive frames, can help identify potential forged areas. Synchronization between audio and video content is another critical factor to verify whether a video has been edited.
Representation Learning:
This method leverages deep learning models to automatically learn effective feature representations from large-scale video datasets. Compared to representation learning in image forgery detection, video forgery detection must pay particular attention to temporal dimensions and motion continuity. By combining 3D convolutional neural networks (CNNs) and recurrent neural networks (RNNs), it becomes possible to capture both spatial and temporal features in videos, enabling effective detection of forgery clues. Additionally, multimodal learning and cross-modal consistency verification techniques are employed to ensure synchronization between visual and auditory content in videos, further enhancing the precision of forgery detection.
Feature Association Analysis Based on Knowledge Graphs
Knowledge graph-based AIGC feature association analysis, as a graph-structured data model, utilizes deep learning, feature extraction, and fingerprint recognition technologies to structurally describe relationships between entities (such as people, events, and locations) and uncover potential complex connections between different data objects. This approach not only effectively identifies potential fraud groups involved in AIGC-generated content but also reveals behavioral patterns and links among multiple fraudulent individuals.
By constructing knowledge graphs based on AIGC features, community detection algorithms can analyze high-density groups of nodes within the graph, identifying individuals belonging to the same fraud group. These nodes often share similar characteristics or behaviors, such as using the same AIGC generation tools or displaying similar forgery features. Association inference helps identify suspicious behavior patterns, uncovering consistent behavioral traits across multiple nodes within the same community, such as frequent use of identical audio forgery or face-swapping techniques.
Furthermore, group expansion and path tracing can reveal the propagation paths of fraud networks by tracking relationships between nodes. For instance, by identifying nodes associated with multiple fake accounts, potential group members can be further uncovered, thereby revealing the complete fraud network. This knowledge graph-based analysis method provides robust technical support for precise targeting of AIGC-related fraud.
The**Financial AIGC Audio-Video Anti-Fraud White Paper(Click here to download)**is divided into seven chapters, primarily covering the risks of audio-video fraud posed by AIGC, typical attack methods of AIGC audio-video fraud, its impact on financial business, anti-fraud solutions for AIGC audio-video, technical implementations for combating AIGC audio-video fraud, typical application scenarios, and outlook and recommendations.