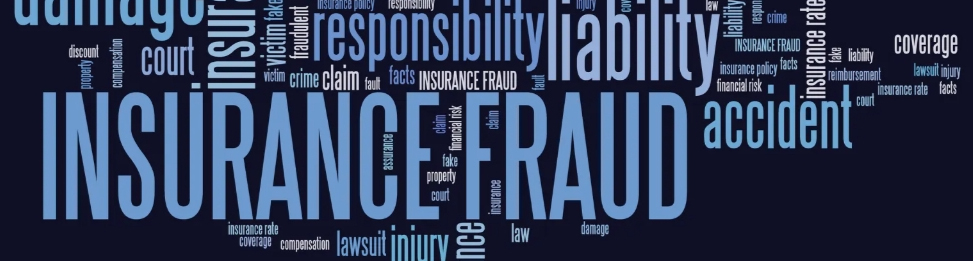
Insurance fraud has long been one of the significant challenges facing the insurance industry, especially with technological advancements enabling fraudsters to continually update their methods. They exploit AI technologies, including generative models, machine learning, and data analytics tools, to deceive insurance companies. The application of AI has become their new tool, making their criminal activities more covert and complex, challenging the anti-fraud measures of the insurance industry.
1. Voice Cloning
: Fraudsters obtain voice samples from social media and use AI technology to generate believable voice clones. These clones can be used to impersonate clients and communicate with insurance agents, making false claim requests.
2. DEEPFAKE Photos and Videos:
Fraudsters use AI technology to create fake photos and videos, conducting fraudulent activities through social networks or video calls. These DEEPFAKE media make it harder for insurance company personnel to identify them.
3. Fabricated Medical Evidence:
AI-driven image generators can create realistic medical evidence such as CT scans or X-rays. This false evidence can be used to submit fraudulent medical claims.
4. Phishing Emails
: Fraudsters use AI robots like ChatGPT to generate realistic phishing attempts and email scam messages to deceive victims.
5. Data Theft for Insurance Applications
: Previously, fraudsters manually filled out false information to submit insurance applications, but now they can automate this process using AI technology. They can extract customer data from multiple sources and automatically fill out application forms. This enables fraudsters to submit a large number of false applications, expanding their fraud scale and making it more challenging to detect.
6. Bypassing Security System
s: Insurance companies typically rely on security systems to detect abnormal data to identify potential fraudulent behavior. However, fraudsters use AI technology, such as manipulation tools for IP addresses and device IDs, to bypass these security triggers. They can quickly switch IP addresses or change device IDs to make it appear as if they are legitimate requests from different sources. This makes it more challenging for insurance companies to detect and prevent fraud.
Automobile Insurance Fraud: Common Types of Fraud
Automobile insurance fraud is one of the most prevalent and costly types of insurance fraud worldwide. In the United States alone, approximately 20% of automobile insurance claims paid out each year fall into the hands of fraudsters, resulting in an average additional expenditure of $400-700 per household annually in premiums.
Automobile insurance fraud can be categorized into soft fraud and hard fraud. Soft fraud typically involves exaggeration of events or claims, such as misrepresenting a minor dent in a bumper caused by scraping a garage wall as a hit-and-run accident. Hard fraud, on the other hand, is usually more severe and involves larger payouts, such as staging accidents or falsely claiming vehicle theft. Specifically, they include:
False Claims:
Individuals may make false or exaggerated claims for losses or damages that did not actually occur under automobile, property, or health insurance.
Staged Accidents:
Some individuals intentionally cause accidents or collisions to fraudulently claim compensation for bodily injury or property damage.
Identity Theft:
Fraudsters may use stolen identities to apply for insurance policies or make claims on behalf of others.
Arson
: Arson is a common form of fraud in property insurance, where policyholders intentionally set fire to property to claim insurance money.
Exaggerated Losses
: Policyholders may exaggerate the extent of their losses when filing claims to seek payouts beyond reasonable costs.
To address these challenges, insurance companies need to continuously enhance technological preventive measures and improve the accuracy and efficiency of fraud detection. Additionally, cooperation with other industries is necessary to collectively combat insurance fraud.
Insurance fraud is an evolving problem, and the advent of artificial intelligence technology has made it more complex and widespread. To effectively address this challenge, insurance companies must continually improve their anti-fraud technologies and utilize advanced data analytics and machine learning algorithms to identify and prevent potential fraudulent activities. At the same time, regulatory bodies and industry associations need to strengthen oversight and cooperation in combating insurance fraud to collectively address this increasingly serious issue.
Insurance Company: Effective Defense Strategies
The Intelligence Special Issue on "DEEPFAKE Threat Research and Security Strategies" suggests that combating AI fraud requires not only effectively identifying and detecting AI-generated content but also preventing the exploitation and proliferation of AI fraud. This requires not only technological countermeasures but also complex psychological warfare and an increase in public safety awareness.
1. Data Analysis and Pattern Recognition
: Data analysis and pattern recognition can help insurance companies identify potential anomalies and behaviors, thereby improving the accuracy of fraud detection. By utilizing AI technology to analyze large amounts of data, abnormal patterns and behaviors can be identified. Monitoring customer historical data and behavior patterns can facilitate the detection of potential fraudulent activities.
2. Behavioral Identification and Analysis:
Machine learning algorithms can analyze customer behavior patterns, including purchasing behavior, claims history, and interactions with the insurance company. Anomalies in behavior may indicate the presence of fraud. Behavioral analysis can uncover abnormal customer behavior, providing vital clues for fraud detection.
Dingxiang Device Fingerprinting bridges internal information of multi-terminal devices to generate unified and unique device fingerprints for each device. It constructs a multi-dimensional identification strategy model based on devices, environments, and behaviors to identify risk devices such as virtual machines, proxy servers, and emulators manipulated maliciously. It analyzes whether there are abnormal behaviors such as multiple account logins, frequent IP address changes, and frequent device attribute changes that do not conform to user habits, tracks and identifies fraudulent activities, helps enterprises achieve operation of the same ID in all scenarios and channels, and assists in cross-channel risk identification and control.
3. Identity Verification:
Adopting biometric technology or other advanced identity verification methods to ensure the authenticity of customer identities, thereby reducing the risks of identity theft and impersonation.
Dingxiang atbCAPTCHA, based on AIGC technology, can prevent threats such as AI brute force attacks, automated attacks, and phishing attacks, effectively preventing unauthorized access, account hijacking, and malicious operations, thereby protecting system stability. It integrates 13 verification methods and various prevention and control strategies, consolidating 4380 risk policies, 112 categories of risk intelligence, covering 24 industries and 118 risk types. Its precision in prevention and control reaches 99.9%, and it can quickly achieve the transformation from risk to intelligence. It also supports secure user passes without perception, and the real-time counter-disposal capability is reduced to within 60 seconds, further improving the convenience and efficiency of digital login services.
4. Image and Facial Recognition
: Analyze images and photos involved in claims to confirm their authenticity. For example, image recognition technology can detect editing traces or signs of modification in images. Image recognition technology can verify the authenticity of images in claims.
Dingxiang's comprehensive panoramic facial security threat perception solution intelligently verifies multidimensional information such as device environment, facial information, image authentication, user behavior, and interaction status, quickly identifying more than 30 types of malicious attacks such as injection attacks, live forgery, image forgery, camera hijacking, debugging risks, memory tampering, Root/jailbreak, malicious ROM, and simulator operations and systems. After discovering forged videos, fake facial images, and abnormal interactive behaviors, it can automatically block operations. It can also flexibly configure video.
5. Natural Language Processing
: Utilize natural language processing technology to analyze claim documents, application forms, and other text information to explore any inconsistent or suspicious language patterns. Natural language processing helps uncover suspicious patterns in textual information.
6. Real-time Monitoring and Warning Systems
: Construct real-time monitoring systems to observe potential fraudulent behavior and take corresponding measures. Warning systems can automatically issue alerts based on predefined rules and patterns. Real-time monitoring and warning systems can promptly detect potential fraudulent activities for intervention.
Dingxiang Dinsight helps enterprises conduct risk assessment, anti-fraud analysis, and real-time monitoring, improving the efficiency and accuracy of risk control. The average processing speed of Dinsigh's daily risk control strategy is within 100 milliseconds, supporting configuration-based access and precipitation of multi-party data. It can realize self-performance monitoring and self-iteration mechanisms based on mature indicators, strategies, models, and deep learning technology. Paired with Dinsight, the Xintell intelligent model platform can automatically optimize security strategies for known risks, support risk control strategies in different scenarios based on risk control logs and data mining potential risks. Based on association network and deep learning technology, it standardizes complex data processing, mining, and machine learning processes, providing one-stop modeling services from data processing, feature derivation, model construction to final model online.
7. Collaborative Anti-fraud Networks:
Build partnerships with other insurance companies, financial institutions, and government agencies to share information and experiences, jointly combatting fraudulent activities. Collaborative anti-fraud networks enhance fraud prevention capabilities through information sharing and collaborative work.
By employing these methods and building a multi-channel, full-scenario, multi-stage security system, effectively combating new threats brought by AI, insurance companies can more efficiently prevent fraudulent risks and safeguard their interests and those of their customers.