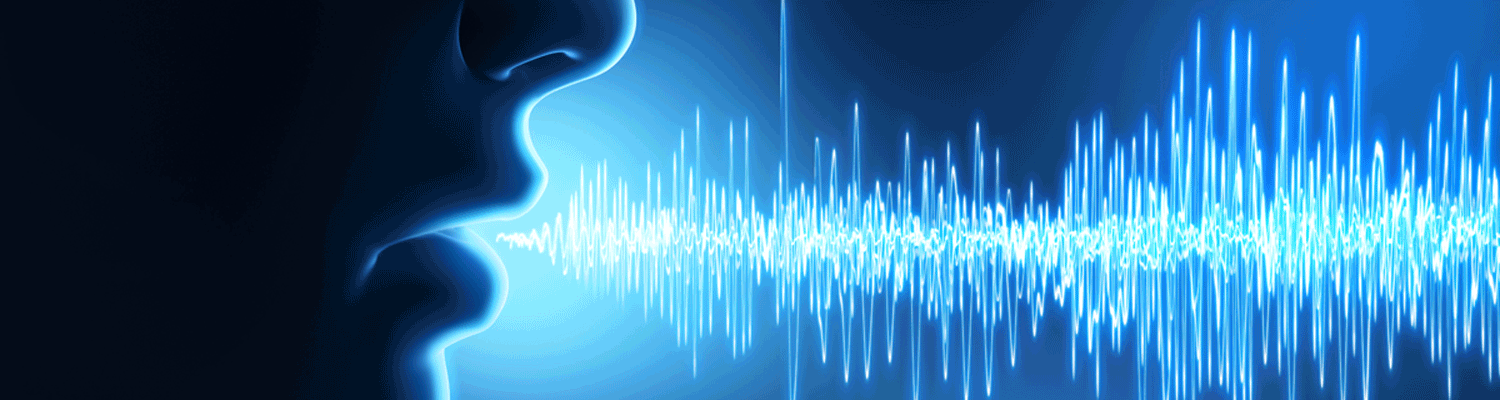
An office worker received an urgent call during a busy workday. The caller claimed to be his boss, informing him that he forgot to transfer funds to a new partner before leaving and needed to handle it immediately. The voice sounded very realistic, and the urgent content made him accept the transfer task without hesitation. After the call ended, he completed the transfer according to the provided wire transfer information. However, it was a scam call based on voice cloning.
The key behind this phone fraud is AI technology. Through advanced speech synthesis and deep learning algorithms, scammers generate extremely realistic voice samples that are almost indistinguishable from the real thing. These voice samples not only mimic a person's tone and intonation but also replicate their language habits and expression styles, making it difficult for the recipient to doubt their authenticity.
The application of voice cloning in phone scams shows that traditional identity verification methods are no longer secure and reliable. Faced with this challenge, technical experts and enterprises need to take multi-layered defense measures: on the one hand, they need to effectively detect and intercept forged voices; platforms need to strengthen the identification of voice cloning; on the other hand, they must prevent the use and spread of voice cloning and verify user behavior and identity; thereby effectively reducing fraud actions by attackers.
Several Technologies for Identifying Voice Cloning
Using advanced AI technology to authenticate the authenticity of voices, timely detecting, and intercepting suspicious content. Developing and applying AI tools for voice anti-counterfeiting, such as using voiceprint recognition technology to confirm the authenticity of the voice, providing security for voice communication.
1. Resemble's Detect-2B
Traditionally, most AI-generated audio clips sound "too clean," lacking the natural noise of real recordings. AI-based models can make judgments by focusing on these subtle differences.
Resemble recently launched the Detect-2B model, an AI solution specifically designed to detect cloned audio. The model architecture is based on Mamba-SSM or state space models, opting not to rely on static data or repetitive patterns but instead using random or probabilistic models. It responds better to different variables and dynamics, maintaining efficiency and accuracy even in poor recording conditions. It effectively handles audio signal diversity, capturing dynamic changes in audio clips, and thus effectively detecting voice cloning.
2. Meta's AudioSeal
Meta's Fundamental AI Research (FAIR) team introduced AudioSeal, an audio watermarking technology designed specifically for detecting AI-generated speech. AudioSeal's core technologies include advanced audio feature extraction and comparison algorithms, as well as efficient audio processing and watermark embedding techniques. Through these technical means, AI models can accurately identify and mark AI-generated audio segments, making the detection process faster and more efficient. Reportedly, the new localized detection method increased detection speed by 485 times, greatly enhancing the capability to handle large-scale audio data.
Compared to traditional methods, AudioSeal can accurately locate AI-generated speech segments in longer audio clips, effectively preventing cloning or tampering.
3. McAfee's Project Mockingbird
McAfee developed the AI model Project Mockingbird, specifically for detecting and identifying AI-generated audio content. Project Mockingbird uses advanced neural network architecture and large-scale data training. The model can accurately analyze audio features, distinguishing AI-generated audio from real recordings. It has rapid response capabilities, allowing real-time detection and identification during audio streaming, effectively addressing immediate audio fraud risks.
4. Multi-Factor Authentication Technology
Based on user behavior and identity to identify attackers. Platforms should analyze user behavior patterns and identity information to establish security warning mechanisms, monitor and restrict suspicious behaviors such as abnormal logins and high-frequency message sending. They should also analyze user behavior patterns like mouse movement and typing style to identify abnormalities, flagging suspicious activities deviating from normal usage. Additionally, extra identity and device verification can be employed. Large models can quickly sift through massive data and identify subtle inconsistencies usually undetectable by humans, discovering abnormal operations by attackers.
Dingxiang Device Fingerprinting can generate a unified and unique device fingerprint for each device, identifying risk devices like virtual machines, proxy servers, and emulators manipulated maliciously. It analyzes whether the device has multiple account logins, frequently changes IP addresses, or frequently changes device attributes to detect abnormal or user-unusual behaviors, tracking and identifying fraudsters' activities.
Dingxiang atbCAPTCHA, based on AIGC technology, can prevent brute force cracking, automated attacks, and phishing attacks by AI, effectively preventing unauthorized access, account hijacking, and malicious operations, thus protecting system stability.
Dingxiang Dinsight Real-Time Risk Control Engine helps enterprises with risk assessment, anti-fraud analysis, and real-time monitoring, improving risk control efficiency and accuracy. Paired with the Xintell Smart Model Platform, it can automatically optimize security strategies for known risks, mine potential risks based on risk control logs and data, and configure risk control strategies for different scenarios with one click.
Voice cloning technology presents both opportunities and threats. The development of effective detection and analysis technologies, as well as comprehensive multi-factor authentication mechanisms, are crucial in mitigating the risks associated with AI-generated voice fraud.